In this article
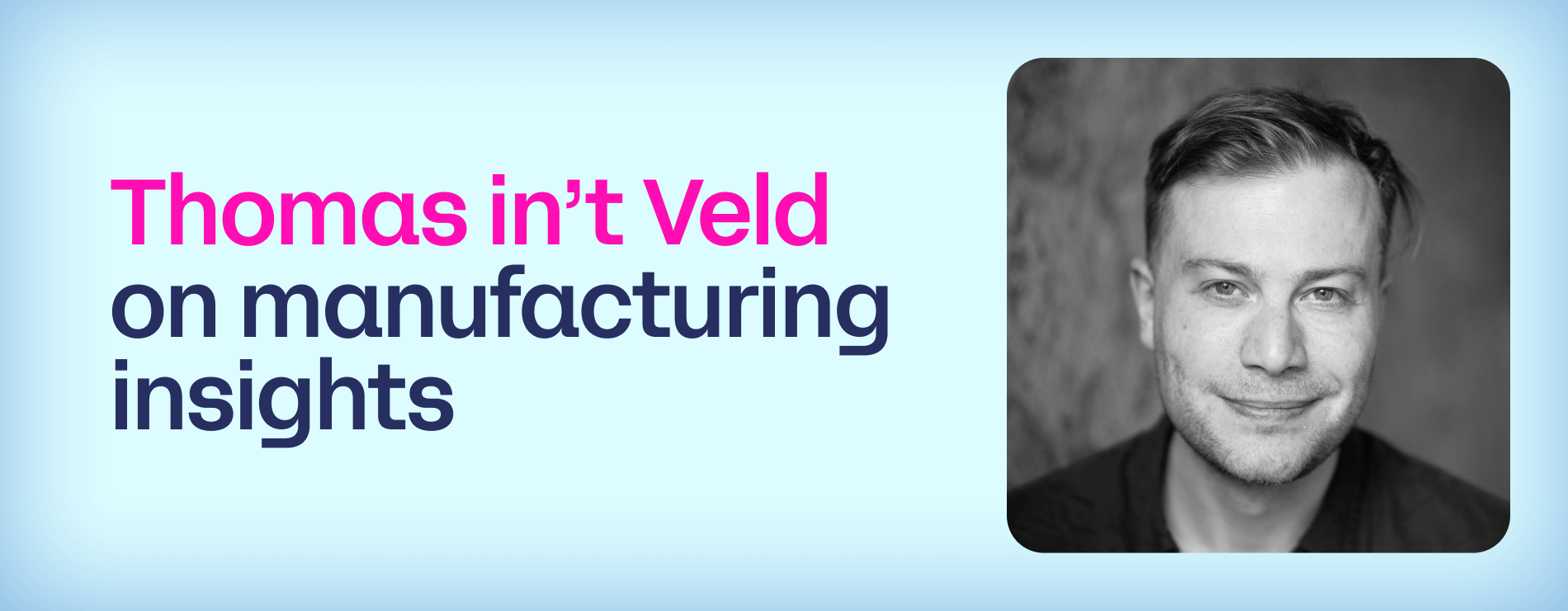
Are you manufacturing insights - or just collecting data?
Data isn't something you just collect — it's something you intentionally produce. This manufacturing mindset is essential for organisations that really want to build a data driven culture (that’s all of you, right?).
Guest post by Thomas in’t Veld, CEO and co-founder of Tasman Analytics. Thomas and his team help companies scale their data practices by applying product thinking to analytics.
Throughout my 10+ years of getting organizations up to speed with great analytics capabilities, I’ve observed that while most companies have rigorous processes for product development, for some reason they treat data and analytics as an afterthought. And the result? Missed opportunities and wasted resources.
This disconnect is quite fundamental. And it explains why so many data initiatives underdeliver despite significant investment. When we do treat data as something to be manufactured with care and intention, we fundamentally shift how we approach analytics within our organisations - and get to that sunlit upland of data-driven culture!
What’s the Problem? Your Ad-hoc Approach to Data
Most organisations work backwards in their data approach. They collect whatever seems available, overdesign the data stack, hope insights emerge naturally, and then scramble when critical decisions need better data than what's on hand.
This results in a cycle of distrust and endless data cleanup that prevents teams from focusing on high-value analysis. Even the best data teams we know still struggle with legacy data model code for this precise reason.
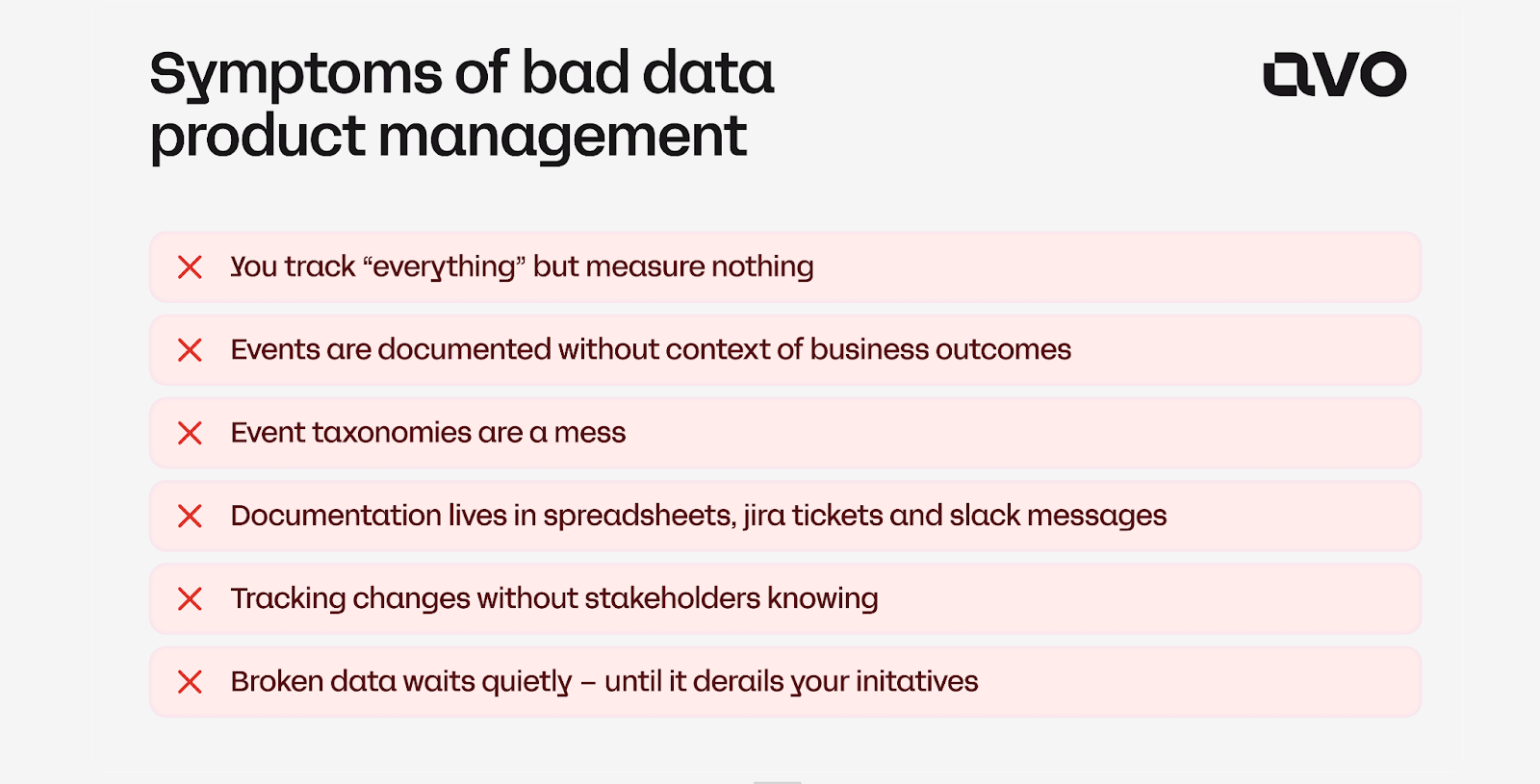
The Avo team has described this as symptoms of bad (or no) data product management in the “Wild West” stage of data maturity. That’s where teams just collect data to satisfy their own short-term needs - without consideration for broader business objectives or future requirements.
Product Design Principles for Data Teams
Let’s be concrete. What does it take to build a data practice that scales, earns trust, and delivers value? And where do you start?
1. Start with User Needs, Not Data Availability
Instead of asking "What data can we collect?" start with "Who needs what information to make which decisions?" This is just a simple reframing, but it transforms how you approach data collection and architecture.
A fintech scale-up we worked with reduced their dashboard metrics from 40 to just 4 key indicators after applying this principle (with a number of dimensions added for reporting granularity). The result wasn't less insight—it was a massive increase in dashboard usage and faster, more confident decision-making throughout the organisation.
2. Create a Product Brief Before Collecting a Single Datapoint
It’s important to have a framework for tracking the right product metrics. At Tasman, we write a product brief for every single data application we build - no matter whether it’s a dashboard, an ad-hoc analysis, or a predictive framework. This briefing is a document that includes primary and secondary users, key decisions the data will inform, quality requirements, and success metrics.
It’s really inspired by product thinking - forcing us to take a step back and think about ownership, applications, and what great looks like.
The process to get here has major business value as well: it transforms vague requests like "we need a dashboard for X" into concrete specifications with clear expectations like “marketing needs to see CAC by cohort, campaign and geo every day in order to make sub-48 hour optimisation decisions”. By treating each data initiative as a product with defined users and goals, we avoid the "collect now, figure out why later" trap.
3. Prioritise Ruthlessly
Not all data is equally valuable — and successful data teams focus their resources on highest-impact use cases. A retail client of ours cut their pipeline cost by 70% while simultaneously increasing actionable insights by focusing exclusively on data directly tied to their growth metrics. And even if you know you want to get to full coverage, focussing on the highest yielding metrics first is always the best start (ie. a 5% increase in marketing efficiency might just about tip the ROI scales from negative into positive).
The best data teams say "no" more than they say "yes." They understand that each additional metric or event comes with maintenance costs and potential for confusion.
(Just an extra thought here. Being great at metrics prioritisation also makes you a GREAT internal consultant for your organisation’s growth. Don’t hold back from sharing strategic thoughts about the business; as an analyst you’re probably the only person really understanding all the moving parts!)
4. Integrate Proper Feedback Loops
Data products need ongoing refinement just like customer-facing products. Implement mechanisms to track usage patterns of your reports and dashboards, and schedule regular review cycles with stakeholders to ensure continued alignment with business needs.
Without these feedback mechanisms, even well-designed data products gradually drift from business reality, leading to decreased trust and usage over time. Pay attention to observability and testing - build them in from the start (and apply more engineering principles here!).
5. Design for Scale
Thoughtful schema design pays enormous dividends down the road. Whether you’re adopting purpose-built tools like Avo or building internal solutions, having systems that support structured design and enforce standards makes all the difference. One of our transportation clients leveraged their initial event structure to build machine learning models two years later without requiring painful data rework—all because they designed with future scale in mind from day oneAnd that’s not to mention the incredible importance of well-structured, documented, accurate datasets as a gold-plated data source for AI Agents.
Let’s Create Your First Data Product Brief!
A small, lightweight process is good for clarity. We typically start with this quick-start approach:
- Identify decision-makers: Who will use this data to make decisions?
- Map decision points: What specific choices will they make with this information?
- Define success metrics: How will you know the data product is delivering value?
- Set quality standards: What level of accuracy, freshness, and completeness is required?
- Establish clear ownership: Who is responsible for each aspect of the data product?
Example: How a Data Product Brief Looks in Practice
Here's a simplified example of how a data product brief might look for an account health scoring project:
[[calloutBlock=Product brief: Account Health Score
INTENT: Define an account health score to support creating a subsequent playbook to generate more value from accounts based on their engagement, intent, and growth potential.
Primary users:
- Marketing team (for campaign segmentation)
- Sales team (for prioritising accounts)
- Customer Success (for proactive retention)
- Product team (for feature development prioritisation)
Key Metrics Framework:
- Product engagement (40%): Measures how users interact with the platform
- Customer engagement (40%): Captures intent signals and interactions
- Growth potential (20%): Evaluates potential for expansion
Success Criteria:
- 90% faster implementation of data-driven campaigns
- Reduction in data quality issues by 80%
- Increased ability to identify at-risk accounts before churn
As you can see, this really does not have to be complex. Instead, strip it down to its essence - tight definitions, clear ownership, and strong success criteria.
]]
Treating data as a product rather than a byproduct fundamentally changes how value is created from your organisation's data assets. It brings the discipline of product development to what is often an ad-hoc process, ensuring that every data initiative has a clear purpose, users, and outcomes.
Start small: select one decision area and create a proper data product brief. The shift in thinking—from collection to manufacturing—may be subtle, but the impact on your data quality and business outcomes will be profound. Let me know how you get on, and don’t hesitate to reach out with comments, observations, or questions!
[[gatedContent]]
Block Quote
Subscribe to our newsletter
White paper: Data Mesh for Event Based Data
Recipe to mature data cultures from wild west to data quality at scale.