In this article
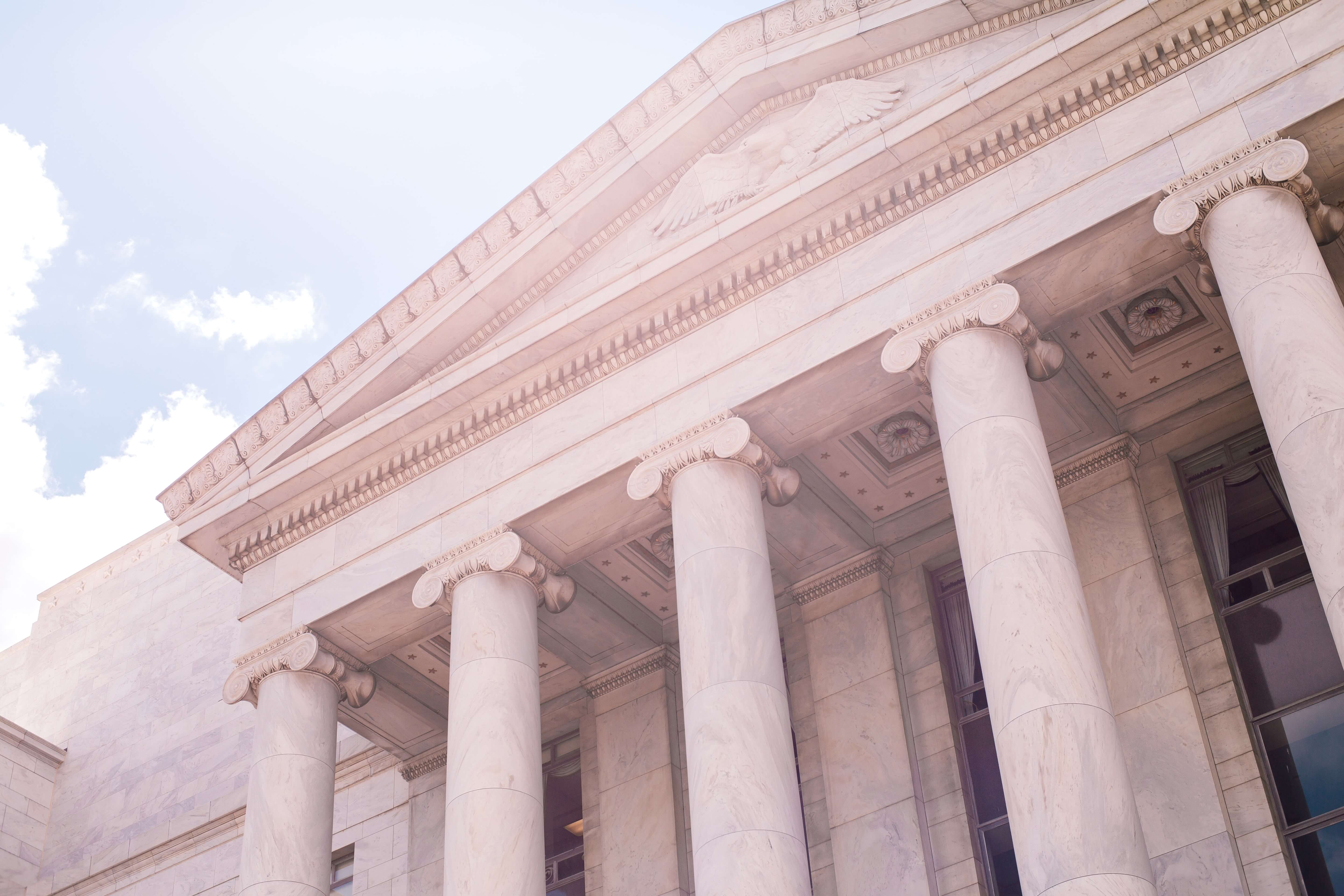
Data Governance Maturity Model: How Mature Is Your Approach to Data?
How mature is your data? Is it a fully-grown partner that helps make your company more profitable and more efficient, or a data infant that muddies up your operations as its fed by messy governance? Find out why you should care about data governance maturity--and how to measure it.
There’s been a global shift toward data-driven business. But, in many cases, the data just under the surface is dirty, and it’s failing modern companies.
In their 2020 Global data management research report, Experian estimated that many businesses aiming for agile, data-driven approaches believe almost a third of their data to be inaccurate.
“While the business wants to be agile and informed by data, this level of distrusted data often leads leaders to fall back on making decisions by gut instinct rather than by informed data insight.” Experian Global Managing Director of Data Quality Mike Kilander
That doesn’t just mean a large chunk of the data the companies have isn’t working for them. It means their data is working against them—costing them money, frustrating their employees, and getting in the way of opportunities.
If you don’t take an active role in your data governance, your dirty data will snowball, picking up crud as it rolls forward. If left uncleaned, it can tarnish up to 70% of your data after one year. This data erosion will directly impact your bottom line--as it does for 83% of companies--and cost you an average of 23% of your revenue.
Mature data governance is no longer a wish list item for modern companies. It’s table stakes, so much so that, according to the 2019 State of Data Management Report, governance is one of the top five strategic initiatives for global companies.
To stop throwing your time and money out the window and make your data clean up and grow up, you need a mature, enterprise-wide data governance model.
These maturity models help companies ensure the data in their system is accurate, well understood, and supported by employees with the skill sets needed to turn numbers into narrative.
What Exactly Is Data Governance Maturity and Why Should You Care?
Data governance maturity refers to the level of data management process and optimization you have built into your business.
At a company with mature data governance, clean and formal data management is at the core of everything they do. Each employee, team lead, and department knows the best practices they’re supposed to follow, and the company’s projects are regularly measured and optimized using the data produced. This gold standard of a data-driven company actively optimizes its processes using the three main components of data governance:
- Rules that dictate the creation and maintenance of data
- Enforcement that outlines how you ensure rules are followed throughout the company
- Management practices that are carried out by data stewards and employees in accordance with enforcement
In its infancy, data governance maturity looks like reactive data management, ad-hoc data practices, a lack of formally defined best practices, and a kind of free-for-all approach to how data is collected, cleaned, and pruned. In short: it looks like chaos, where data is a byproduct of your company instead of the fuel behind its actions.
If you aren’t taking a proactive role in your data governance by measuring its maturity, you’re letting an information toddler run through an operational china shop—a dirty-data toddler that can wreck your company, leaving errors, inconsistencies, and security risks in its path.
“Poor data is often entangling entire organizations with crippling effects, preventing timely decision-making and negatively impacting customer experience.” — Experian 2020 Global data management research report
However, there’s no “one size fits all” approach to data governance, so you’ll have to work with your leaders and systems architects to tailor the framework that fits your use case and goals.
Which Data Governance Maturity Models Should You Use?
Data governance maturity models help you measure and understand how well you manage your data. When you have a clear understanding of your data governance, you’re better able to understand how you are or are not meeting goals, what steps you need to take to improve data management overall, and how you need to tailor data approaches to each team and project.
There are almost as many data governance maturity models as there are use cases for data. If you already use a maturity model to measure your data capabilities, it’s best to adjust that framework and apply it to your data governance to keep things consistent.
If, however, you’re starting from the ground level, you can choose from one of the many maturity assessments published by reputable vendors, each of which features a slightly different emphasis:
- IBM & Deloitte focus on process characterization across projects and enterprise levels and use the CMMI Institute’s Data Management Maturity (DMM) model.
- DataFlux focuses on people, risk, process, reward, technology adoption, and business capabilities.
- EDM Council focuses on the maturity of an organization’s structure, its policies and guidelines, and funding.
- Kalido focuses on the value of data as an enterprise asset and the risks and rewards of how it’s managed.
All of the above feature some version of the level 1-5 system to rate the development stage of a company’s data governance.
For this article, we’ll focus on the IBM model, as the Capability Maturity Model Integration (CMMI) framework that underpins it is one of the most popular—and oldest—ways of thinking about data governance maturity.
The Capability Maturity Model Integration (CMMI) was developed in 2006 by the Software Engineering Institute at Carnegie Mellon University based on the earlier Capability Maturity Model (CMM). The CMM was originally developed to improve software dev processes, and its successor took its original principles and made them more broadly applicable to any enterprise using data.
IBM Data Governance Maturity Model
The IBM Data Governance Maturity Model is one of the most widely used models around for measuring the maturity of data governance. Drawing from the creation of the CMMI in 2006, IBM released this model in 2007 to provide a clear, easy-to-understand, and tailored framework for measuring data maturity.
The IBM Data Governance Maturity Model focuses on measuring 11 domains—such as value creation, policy, stewardship, information lifecycle management, and data architecture—using a maturity scale of 1-5. Most companies start out by focusing on the handful that are most relevant and important to them and then building out as their core domains become more mature.
The 5 levels of maturity
For each domain a company focuses on, the IBM Data Governance Maturity Model outlines five levels of maturity.

Below, we’ll take a look at what exactly maturity at each level means and how you can level up to the next stage based on your current data governance practices.
Level 1: Initial
Companies with level 1 data governance have little to no data management processes in place, are reactive to data management, and have no formalized tracking plan.
How to Uplevel: In order to move beyond level 1, system architects should audit information flow and develop a plan for managing it with stakeholders and IT managers.
Level 2: Managed
Companies that achieve level 2 data governance have slightly more processes in place, some automation integrated, and more awareness of data. This is where most companies start their data governance journey.
How to Uplevel: To upgrade your data governance maturity to level 3, focus on developing data regulation and documentation guidelines. Your system architects should begin to do this by building models of core infrastructure and documentation needs.
Level 3: Defined
Level 3 data governance means that there are some defined policies in place, you’re regularly leveraging technology for data management, you’ve developed a road map for integrating data, and best practices are more widely understood across the company.
How to Uplevel: As you move into level 4, continue to implement data stewardship by assigning and implementing policies and rules of data management. This policy framework will underpin regular risk assessments and help you create a central program for educating teams on data best practices.
Level 4: Quantitatively Managed
By level 4, your company should have a clear, enterprise-wide data governance structure with projects that follow your set rules and principles and models that are well documented and regularly reviewed. You should have a well-defined policy framework that outlines frequent risk assessments and methods for educating enterprise teams on the company’s data management best practices.
How to Uplevel: Now that your framework has been created and implemented, focus on defining and setting performance measurement metrics for each process. To do this, create a plan for maintaining data models and check that each project follows your data best practices to avoid dirtying data down the line.
Level 5: Optimizing
Level 5 is the holy grail of data governance. 🏆 At this point, data governance should be streamlined, and processes should be automated. Data governance is at the core of how you do business.
This means that data governance is no longer an afterthought or detour on the way to how you do business. Instead, all teams and employees should know how to follow data best practices, and every project you do should follow these rules and principles to create and utilize clean data.
Since this is the ultimate level of data governance, there is no upleveling from here. Instead, if you reach level 5, focus on continuously measuring and analyzing ROI for all projects, so you keep your data integrity.
Make Better Decisions with Better Data
Your data governance is only as mature as the data underneath it is good. To become truly data-driven, you must ensure you have a single, high-quality source of truth to work with.
Avo helps you create this single source of truth and make the right data-driven decisions to move your business by allowing you to prioritize, inspect, analyze, and future-proof your tracking to create reliable data now and tomorrow.
Start using Avo today to create better data practices for tomorrow.
Block Quote